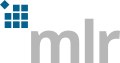
Function reference
-
agri.task
- European Union Agricultural Workforces clustering task.
-
bc.task
- Wisconsin Breast Cancer classification task.
-
bh.task
- Boston Housing regression task.
-
costiris.task
- Iris cost-sensitive classification task.
-
fuelsubset.task
- FuelSubset functional data regression task.
-
gunpoint.task
- Gunpoint functional data classification task.
-
iris.task
- Iris classification task.
-
lung.task
- NCCTG Lung Cancer survival task.
-
mtcars.task
- Motor Trend Car Road Tests clustering task.
-
phoneme.task
- Phoneme functional data multilabel classification task.
-
pid.task
- PimaIndiansDiabetes classification task.
-
sonar.task
- Sonar classification task.
-
spam.task
- Spam classification task.
-
spatial.task
- J. Muenchow's Ecuador landslide data set
-
wpbc.task
- Wisonsin Prognostic Breast Cancer (WPBC) survival task.
-
yeast.task
- Yeast multilabel classification task.
-
Task
- Create a classification, regression, survival, cluster, cost-sensitive classification or multilabel task.
-
makeClassifTask()
- Create a classification task.
-
makeClusterTask()
- Create a cluster task.
-
makeCostSensTask()
- Create a cost-sensitive classification task.
-
makeMultilabelTask()
- Create a multilabel task.
-
makeRegrTask()
- Create a regression task.
-
makeSurvTask()
- Create a survival task.
-
convertMLBenchObjToTask()
- Convert a machine learning benchmark / demo object from package mlbench to a task.
-
getFunctionalFeatures()
- Get only functional features from a task or a data.frame.
-
listLearners()
- Find matching learning algorithms.
-
listMeasures()
- Find matching measures.
-
subsetTask()
- Subset data in task.
-
TaskDesc
- Description object for task.
-
listTaskTypes()
- List the supported task types in mlr
-
train()
- Train a learning algorithm.
-
trainLearner()
- Train an R learner.
-
TuneControl
- Control object for tuning
-
makeTuneMultiCritControlGrid()
makeTuneMultiCritControlMBO()
makeTuneMultiCritControlNSGA2()
makeTuneMultiCritControlRandom()
- Create control structures for multi-criteria tuning.
-
TuneMultiCritResult
- Result of multi-criteria tuning.
-
TuneResult
- Result of tuning.
-
makeTuneControlCMAES()
- Create control object for hyperparameter tuning with CMAES.
-
makeTuneControlDesign()
- Create control object for hyperparameter tuning with predefined design.
-
makeTuneControlGenSA()
- Create control object for hyperparameter tuning with GenSA.
-
makeTuneControlGrid()
- Create control object for hyperparameter tuning with grid search.
-
makeTuneControlIrace()
- Create control object for hyperparameter tuning with Irace.
-
makeTuneControlMBO()
- Create control object for hyperparameter tuning with MBO.
-
makeTuneControlRandom()
- Create control object for hyperparameter tuning with random search.
-
tuneParams()
- Hyperparameter tuning.
-
tuneParamsMultiCrit()
- Hyperparameter tuning for multiple measures at once.
-
tuneThreshold()
- Tune prediction threshold.
-
removeHyperPars()
- Remove hyperparameters settings of a learner.
-
predict(<WrappedModel>)
- Predict new data.
-
asROCRPrediction()
- Converts predictions to a format package ROCR can handle.
-
predictLearner()
- Predict new data with an R learner.
-
makeRLearner()
makeRLearnerClassif()
makeRLearnerMultilabel()
makeRLearnerRegr()
makeRLearnerSurv()
makeRLearnerCluster()
makeRLearnerCostSens()
- Internal construction / wrapping of learner object.
-
listLearners()
- Find matching learning algorithms.
-
learners
- List of supported learning algorithms.
-
makeLearner()
- Create learner object.
-
listLearnerProperties()
- List the supported learner properties
-
makeLearners()
- Create multiple learners at once.
-
learnerArgsToControl()
- Convert arguments to control structure.
-
makeRLearner(<classif.fdausc.glm>)
- Classification of functional data by Generalized Linear Models.
-
makeRLearner(<classif.fdausc.kernel>)
- Learner for kernel classification for functional data.
-
makeRLearner(<classif.fdausc.np>)
- Learner for nonparametric classification for functional data.
-
makeStackedLearner()
- Create a stacked learner object.
-
resample()
crossval()
repcv()
holdout()
subsample()
bootstrapOOB()
bootstrapB632()
bootstrapB632plus()
growingcv()
fixedcv()
- Fit models according to a resampling strategy.
-
ResamplePrediction
- Prediction from resampling.
-
ResampleResult
- ResampleResult object.
-
makeCustomResampledMeasure()
- Construct your own resampled performance measure.
-
makeResampleDesc()
- Create a description object for a resampling strategy.
-
makeResampleInstance()
- Instantiates a resampling strategy object.
-
makeFixedHoldoutInstance()
- Generate a fixed holdout instance for resampling.
-
batchmark()
- Run machine learning benchmarks as distributed experiments.
-
parallelization
- Supported parallelization methods
-
createDummyFeatures()
- Generate dummy variables for factor features.
-
downsample()
- Downsample (subsample) a task or a data.frame.
-
dropFeatures()
- Drop some features of task.
-
generateCalibrationData()
- Generate classifier calibration data.
-
capLargeValues()
- Convert large/infinite numeric values in a data.frame or task.
-
crossover
- Crossover.
-
joinClassLevels()
- Join some class existing levels to new, larger class levels for classification problems.
-
mergeSmallFactorLevels()
- Merges small levels of factors into new level.
-
normalizeFeatures()
- Normalize features.
-
oversample()
undersample()
- Over- or undersample binary classification task to handle class imbalancy.
-
removeConstantFeatures()
- Remove constant features from a data set.
-
summarizeColumns()
- Summarize columns of data.frame or task.
-
summarizeLevels()
- Summarizes factors of a data.frame by tabling them.
-
smote()
- Synthetic Minority Oversampling Technique to handle class imbalancy in binary classification.
-
benchmark()
- Benchmark experiment for multiple learners and tasks.
-
BenchmarkResult
- BenchmarkResult object.
-
makeModelMultiplexer()
- Create model multiplexer for model selection to tune over multiple possible models.
-
makeModelMultiplexerParamSet()
- Creates a parameter set for model multiplexer tuning.
-
mergeBenchmarkResults()
- Merge different BenchmarkResult objects.
-
reduceBatchmarkResults()
- Reduce results of a batch-distributed benchmark.
-
convertBMRToRankMatrix()
- Convert BenchmarkResult to a rank-matrix.
-
friedmanPostHocTestBMR()
- Perform a posthoc Friedman-Nemenyi test.
-
friedmanTestBMR()
- Perform overall Friedman test for a BenchmarkResult.
-
getBMRAggrPerformances()
- Extract the aggregated performance values from a benchmark result.
-
getBMRFeatSelResults()
- Extract the feature selection results from a benchmark result.
-
getBMRFilteredFeatures()
- Extract the feature selection results from a benchmark result.
-
getBMRLearnerIds()
- Return learner ids used in benchmark.
-
getBMRLearnerShortNames()
- Return learner short.names used in benchmark.
-
getBMRLearners()
- Return learners used in benchmark.
-
getBMRMeasureIds()
- Return measures IDs used in benchmark.
-
getBMRMeasures()
- Return measures used in benchmark.
-
getBMRModels()
- Extract all models from benchmark result.
-
getBMRPerformances()
- Extract the test performance values from a benchmark result.
-
getBMRPredictions()
- Extract the predictions from a benchmark result.
-
getBMRTaskDescriptions()
- Extract all task descriptions from benchmark result (DEPRECATED).
-
getBMRTaskDescs()
- Extract all task descriptions from benchmark result.
-
getBMRTaskIds()
- Return task ids used in benchmark.
-
getBMRTuneResults()
- Extract the tuning results from a benchmark result.
-
plotBMRBoxplots()
- Create box or violin plots for a BenchmarkResult.
-
plotBMRRanksAsBarChart()
- Create a bar chart for ranks in a BenchmarkResult.
-
plotBMRSummary()
- Plot a benchmark summary.
-
makeFeatSelControlExhaustive()
makeFeatSelControlGA()
makeFeatSelControlRandom()
makeFeatSelControlSequential()
- Create control structures for feature selection.
-
FeatSelResult
- Result of feature selection.
-
filterFeatures()
- Filter features by thresholding filter values.
-
generateFilterValuesData()
- Calculates feature filter values.
-
getBMRFilteredFeatures()
- Extract the feature selection results from a benchmark result.
-
getFilteredFeatures()
- Returns the filtered features.
-
listFilterEnsembleMethods()
- List ensemble filter methods.
-
listFilterMethods()
- List filter methods.
-
makeFilter()
- Create a feature filter.
-
makeFilterEnsemble()
- Create an ensemble feature filter.
-
makeFilterWrapper()
- Fuse learner with a feature filter method.
-
plotFilterValues()
- Plot filter values using ggplot2.
-
generateFeatureImportanceData()
- Generate feature importance.
-
analyzeFeatSelResult()
- Show and visualize the steps of feature selection.
-
selectFeatures()
- Feature selection by wrapper approach.
-
calculateConfusionMatrix()
print(<ConfusionMatrix>)
- Confusion matrix.
-
estimateRelativeOverfitting()
- Estimate relative overfitting.
-
estimateResidualVariance()
- Estimate the residual variance.
-
generateHyperParsEffectData()
- Generate hyperparameter effect data.
-
generateLearningCurveData()
- Generates a learning curve.
-
generatePartialDependenceData()
- Generate partial dependence.
-
generateThreshVsPerfData()
- Generate threshold vs. performance(s) for 2-class classification.
-
generateCritDifferencesData()
- Generate data for critical-differences plot.
-
performance()
- Measure performance of prediction.
-
measureSSE()
measureMSE()
measureRMSE()
measureMEDSE()
measureSAE()
measureMAE()
measureMEDAE()
measureRSQ()
measureEXPVAR()
measureRRSE()
measureRAE()
measureMAPE()
measureMSLE()
measureRMSLE()
measureKendallTau()
measureSpearmanRho()
measureMMCE()
measureACC()
measureBER()
measureAUNU()
measureAUNP()
measureAU1U()
measureAU1P()
measureMulticlassBrier()
measureLogloss()
measureSSR()
measureQSR()
measureLSR()
measureKAPPA()
measureWKAPPA()
measureAUC()
measureBrier()
measureBrierScaled()
measureBAC()
measureTP()
measureTN()
measureFP()
measureFN()
measureTPR()
measureTNR()
measureFPR()
measureFNR()
measurePPV()
measureNPV()
measureFDR()
measureMCC()
measureF1()
measureGMEAN()
measureGPR()
measureMultilabelHamloss()
measureMultilabelSubset01()
measureMultilabelF1()
measureMultilabelACC()
measureMultilabelPPV()
measureMultilabelTPR()
- Performance measures.
-
Aggregation
- Aggregation object.
-
ConfusionMatrix
- Confusion matrix
-
addRRMeasure()
- Compute new measures for existing ResampleResult
-
aggregations
test.mean
test.sd
test.median
test.min
test.max
test.sum
test.range
test.rmse
train.mean
train.sd
train.median
train.min
train.max
train.sum
train.range
train.rmse
b632
b632plus
testgroup.mean
testgroup.sd
test.join
- Aggregation methods.
-
calculateROCMeasures()
print(<ROCMeasures>)
- Calculate receiver operator measures.
-
makeAggregation()
- Specify your own aggregation of measures.
-
makeMeasure()
- Construct performance measure.
-
makeCostMeasure()
- Creates a measure for non-standard misclassification costs.
-
listMeasureProperties()
- List the supported measure properties.
-
setAggregation()
- Set aggregation function of measure.
-
simplifyMeasureNames()
- Simplify measure names.
-
imputeConstant()
imputeMedian()
imputeMean()
imputeMode()
imputeMin()
imputeMax()
imputeUniform()
imputeNormal()
imputeHist()
imputeLearner()
- Built-in imputation methods.
-
impute()
- Impute and re-impute data
-
makeImputeMethod()
- Create a custom imputation method.
-
reimpute()
- Re-impute a data set
-
createSpatialResamplingPlots()
- Create (spatial) resampling plot objects.
-
extractFDABsignal()
- Bspline mlq features
-
extractFDADTWKernel()
- DTW kernel features
-
extractFDAFPCA()
- Extract functional principal component analysis features.
-
extractFDAFeatures()
- Extract features from functional data.
-
extractFDAFourier()
- Fast Fourier transform features.
-
extractFDAMultiResFeatures()
- Multiresolution feature extraction.
-
extractFDATsfeatures()
- Time-Series Feature Heuristics
-
extractFDAWavelets()
- Discrete Wavelet transform features.
-
makeExtractFDAFeatMethod()
- Constructor for FDA feature extraction methods.
-
makeExtractFDAFeatsWrapper()
- Fuse learner with an extractFDAFeatures method.
-
reextractFDAFeatures()
- Re-extract features from a data set
-
getFunctionalFeatures()
- Get only functional features from a task or a data.frame.
-
hasFunctionalFeatures()
- Check whether the object contains functional features.
-
makeFunctionalData()
- Create a data.frame containing functional features from a normal data.frame.
-
configureMlr()
- Configures the behavior of the package.
-
mlr
mlr-package
- mlr: Machine Learning in R
-
mlrFamilies
- mlr documentation families
-
getMlrOptions()
- Returns a list of mlr's options.
-
plotBMRBoxplots()
- Create box or violin plots for a BenchmarkResult.
-
plotBMRRanksAsBarChart()
- Create a bar chart for ranks in a BenchmarkResult.
-
plotBMRSummary()
- Plot a benchmark summary.
-
plotCalibration()
- Plot calibration data using ggplot2.
-
plotCritDifferences()
- Plot critical differences for a selected measure.
-
plotFilterValues()
- Plot filter values using ggplot2.
-
plotHyperParsEffect()
- Plot the hyperparameter effects data
-
plotLearnerPrediction()
- Visualizes a learning algorithm on a 1D or 2D data set.
-
plotLearningCurve()
- Plot learning curve data using ggplot2.
-
plotPartialDependence()
- Plot a partial dependence with ggplot2.
-
plotROCCurves()
- Plots a ROC curve using ggplot2.
-
plotResiduals()
- Create residual plots for prediction objects or benchmark results.
-
plotThreshVsPerf()
- Plot threshold vs. performance(s) for 2-class classification using ggplot2.
-
plotTuneMultiCritResult()
- Plots multi-criteria results after tuning using ggplot2.
-
makeCostSensTask()
- Create a cost-sensitive classification task.
-
makeCostSensClassifWrapper()
- Wraps a classification learner for use in cost-sensitive learning.
-
makeCostSensRegrWrapper()
- Wraps a regression learner for use in cost-sensitive learning.
-
makeCostSensWeightedPairsWrapper()
- Wraps a classifier for cost-sensitive learning to produce a weighted pairs model.
-
extractFDABsignal()
- Bspline mlq features
-
extractFDADTWKernel()
- DTW kernel features
-
extractFDAFPCA()
- Extract functional principal component analysis features.
-
extractFDAFeatures()
- Extract features from functional data.
-
extractFDAFourier()
- Fast Fourier transform features.
-
extractFDAMultiResFeatures()
- Multiresolution feature extraction.
-
extractFDATsfeatures()
- Time-Series Feature Heuristics
-
extractFDAWavelets()
- Discrete Wavelet transform features.
-
getCacheDir()
deleteCacheDir()
- Get or delete mlr cache directory
-
helpLearner()
- Access help page of learner functions.
-
helpLearnerParam()
- Get specific help for a learner's parameters.
-
FailureModel
- Failure model.
-
hasProperties()
- Deprecated, use
hasLearnerProperties
instead.
-
isFailureModel()
- Is the model a FailureModel?
-
makeBaggingWrapper()
- Fuse learner with the bagging technique.
-
makeClassificationViaRegressionWrapper()
- Classification via regression wrapper.
-
makeConstantClassWrapper()
- Wraps a classification learner to support problems where the class label is (almost) constant.
-
makeCostSensClassifWrapper()
- Wraps a classification learner for use in cost-sensitive learning.
-
makeCostSensRegrWrapper()
- Wraps a regression learner for use in cost-sensitive learning.
-
makeCostSensWeightedPairsWrapper()
- Wraps a classifier for cost-sensitive learning to produce a weighted pairs model.
-
makeDownsampleWrapper()
- Fuse learner with simple downsampling (subsampling).
-
makeDummyFeaturesWrapper()
- Fuse learner with dummy feature creator.
-
makeExtractFDAFeatsWrapper()
- Fuse learner with an extractFDAFeatures method.
-
makeFeatSelWrapper()
- Fuse learner with feature selection.
-
makeFilterWrapper()
- Fuse learner with a feature filter method.
-
makeImputeWrapper()
- Fuse learner with an imputation method.
-
makeMulticlassWrapper()
- Fuse learner with multiclass method.
-
makeMultilabelBinaryRelevanceWrapper()
- Use binary relevance method to create a multilabel learner.
-
makeMultilabelClassifierChainsWrapper()
- Use classifier chains method (CC) to create a multilabel learner.
-
makeMultilabelDBRWrapper()
- Use dependent binary relevance method (DBR) to create a multilabel learner.
-
makeMultilabelNestedStackingWrapper()
- Use nested stacking method to create a multilabel learner.
-
makeMultilabelStackingWrapper()
- Use stacking method (stacked generalization) to create a multilabel learner.
-
makeOverBaggingWrapper()
- Fuse learner with the bagging technique and oversampling for imbalancy correction.
-
makePreprocWrapper()
- Fuse learner with preprocessing.
-
makePreprocWrapperCaret()
- Fuse learner with preprocessing.
-
makeRemoveConstantFeaturesWrapper()
- Fuse learner with removal of constant features preprocessing.
-
makeSMOTEWrapper()
- Fuse learner with SMOTE oversampling for imbalancy correction in binary classification.
-
makeTuneWrapper()
- Fuse learner with tuning.
-
makeUndersampleWrapper()
makeOversampleWrapper()
- Fuse learner with simple ove/underrsampling for imbalancy correction in binary classification.
-
makeWeightedClassesWrapper()
- Wraps a classifier for weighted fitting where each class receives a weight.
-
makeWrappedModel()
- Induced model of learner.
-
getLearnerProperties()
hasLearnerProperties()
- Query properties of learners.
-
getMeasureProperties()
hasMeasureProperties()
- Query properties of measures.
-
getCacheDir()
deleteCacheDir()
- Get or delete mlr cache directory
-
getBMRAggrPerformances()
- Extract the aggregated performance values from a benchmark result.
-
getBMRFeatSelResults()
- Extract the feature selection results from a benchmark result.
-
getBMRFilteredFeatures()
- Extract the feature selection results from a benchmark result.
-
getBMRLearnerIds()
- Return learner ids used in benchmark.
-
getBMRLearnerShortNames()
- Return learner short.names used in benchmark.
-
getBMRLearners()
- Return learners used in benchmark.
-
getBMRMeasureIds()
- Return measures IDs used in benchmark.
-
getBMRMeasures()
- Return measures used in benchmark.
-
getBMRModels()
- Extract all models from benchmark result.
-
getBMRPerformances()
- Extract the test performance values from a benchmark result.
-
getBMRPredictions()
- Extract the predictions from a benchmark result.
-
getBMRTaskDescriptions()
- Extract all task descriptions from benchmark result (DEPRECATED).
-
getBMRTaskDescs()
- Extract all task descriptions from benchmark result.
-
getBMRTaskIds()
- Return task ids used in benchmark.
-
getBMRTuneResults()
- Extract the tuning results from a benchmark result.
-
getCaretParamSet()
- Get tuning parameters from a learner of the caret R-package.
-
getClassWeightParam()
- Get the class weight parameter of a learner.
-
getConfMatrix()
- Confusion matrix.
-
getDefaultMeasure()
- Get default measure.
-
getFailureModelDump()
- Return the error dump of FailureModel.
-
getFailureModelMsg()
- Return error message of FailureModel.
-
getFeatSelResult()
- Returns the selected feature set and optimization path after training.
-
getFeatureImportance()
- Calculates feature importance values for trained models.
-
getFilteredFeatures()
- Returns the filtered features.
-
getFunctionalFeatures()
- Get only functional features from a task or a data.frame.
-
getHomogeneousEnsembleModels()
- Deprecated, use
getLearnerModel
instead.
-
getHyperPars()
- Get current parameter settings for a learner.
-
getLearnerId()
- Get the ID of the learner.
-
getLearnerModel()
- Get underlying R model of learner integrated into mlr.
-
getLearnerNote()
- Get the note for the learner.
-
getLearnerPackages()
- Get the required R packages of the learner.
-
getLearnerParVals()
- Get the parameter values of the learner.
-
getLearnerParamSet()
- Get the parameter set of the learner.
-
getLearnerPredictType()
- Get the predict type of the learner.
-
getLearnerShortName()
- Get the short name of the learner.
-
getLearnerType()
- Get the type of the learner.
-
getMlrOptions()
- Returns a list of mlr's options.
-
getMultilabelBinaryPerformances()
- Retrieve binary classification measures for multilabel classification predictions.
-
getNestedTuneResultsOptPathDf()
- Get the
opt.path
s from each tuning step from the outer resampling.
-
getNestedTuneResultsX()
- Get the tuned hyperparameter settings from a nested tuning.
-
getOOBPreds()
- Extracts out-of-bag predictions from trained models.
-
getParamSet
- Get a description of all possible parameter settings for a learner.
-
getPredictionDump()
- Return the error dump of a failed Prediction.
-
getPredictionProbabilities()
- Get probabilities for some classes.
-
getPredictionResponse()
getPredictionSE()
getPredictionTruth()
- Get response / truth from prediction object.
-
getPredictionTaskDesc()
- Get summarizing task description from prediction.
-
getProbabilities()
- Deprecated, use
getPredictionProbabilities
instead.
-
getRRDump()
- Return the error dump of ResampleResult.
-
getRRPredictionList()
- Get list of predictions for train and test set of each single resample iteration.
-
getRRPredictions()
- Get predictions from resample results.
-
getRRTaskDesc()
- Get task description from resample results (DEPRECATED).
-
getRRTaskDescription()
- Get task description from resample results (DEPRECATED).
-
getResamplingIndices()
- Get the resampling indices from a tuning or feature selection wrapper..
-
getStackedBaseLearnerPredictions()
- Returns the predictions for each base learner.
-
getTaskClassLevels()
- Get the class levels for classification and multilabel tasks.
-
getTaskCosts()
- Extract costs in task.
-
getTaskData()
- Extract data in task.
-
getTaskDesc()
- Get a summarizing task description.
-
getTaskDescription()
- Deprecated, use getTaskDesc instead.
-
getTaskFeatureNames()
- Get feature names of task.
-
getTaskFormula()
- Get formula of a task.
-
getTaskId()
- Get the id of the task.
-
getTaskNFeats()
- Get number of features in task.
-
getTaskSize()
- Get number of observations in task.
-
getTaskTargetNames()
- Get the name(s) of the target column(s).
-
getTaskTargets()
- Get target data of task.
-
getTaskType()
- Get the type of the task.
-
getTuneResult()
- Returns the optimal hyperparameters and optimization path after training.
-
getTuneResultOptPath()
- Get the optimization path of a tuning result.
-
setAggregation()
- Set aggregation function of measure.
-
setHyperPars()
- Set the hyperparameters of a learner object.
-
setHyperPars2()
- Only exported for internal use.
-
setId()
- Set the id of a learner object.
-
setLearnerId()
- Set the ID of a learner object.
-
setMeasurePars()
- Set parameters of performance measures
-
setPredictThreshold()
- Set the probability threshold the learner should use.
-
setPredictType()
- Set the type of predictions the learner should return.
-
setThreshold()
- Set threshold of prediction object.