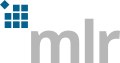
Out-of-Bag Predictions
Source:vignettes/tutorial/out_of_bag_predictions.Rmd
out_of_bag_predictions.Rmd
Some learners like random forest use bagging. Bagging means that the learner consists of an ensemble of several base learners and each base learner is trained with a different random subsample or bootstrap sample from all observations. A prediction made for an observation in the original data set using only base learners not trained on this particular observation is called out-of-bag (OOB) prediction. These predictions are not prone to overfitting, as each prediction is only made by learners that did not use the observation for training.
To get a list of learners that provide OOB predictions, you can call listLearners(obj = NA, properties = "oobpreds")
.
listLearners(obj = NA, properties = "oobpreds")[c("class", "package")]
## class package
## 1 classif.randomForest randomForest
## 2 classif.ranger ranger
## 3 classif.rFerns rFerns
## 4 regr.randomForest randomForest
## 5 regr.ranger ranger
In mlr
function getOOBPreds()
can be used to extract these observations from the trained models. These predictions can be used to evaluate the performance of a given learner like in the following example.
lrn = makeLearner("classif.ranger", predict.type = "prob", predict.threshold = 0.6)
mod = train(lrn, sonar.task)
oob = getOOBPreds(mod, sonar.task)
oob
## Prediction: 208 observations
## predict.type: prob
## threshold: M=0.60,R=0.40
## time: NA
## id truth prob.M prob.R response
## 1 1 R 0.5386034 0.4613966 R
## 2 2 R 0.5097282 0.4902718 R
## 3 3 R 0.5730803 0.4269197 R
## 4 4 R 0.3708941 0.6291059 R
## 5 5 R 0.5461197 0.4538803 R
## 6 6 R 0.4172523 0.5827477 R
## ... (#rows: 208, #cols: 5)
performance(oob, measures = list(auc, mmce))
## auc mmce
## 0.9332219 0.1923077
As the predictions that are used are out-of-bag, this evaluation strategy is very similar to common resampling strategies like 10-fold cross-validation, but much faster, as only one training instance of the model is required.